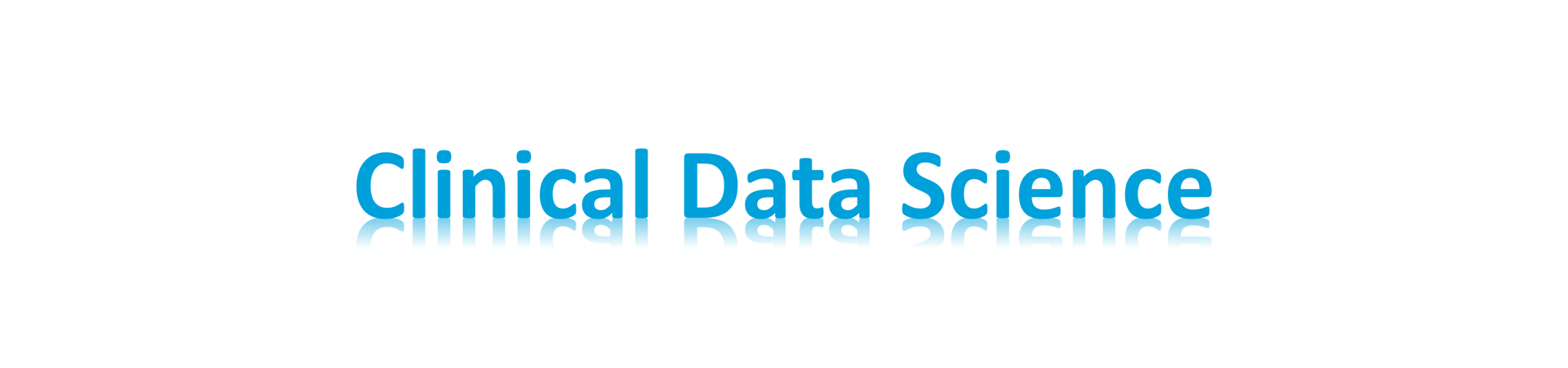
Clinical Data Science
BackModern clinical data science seeks to develop a new generation of quantitative methods with which to predict clinical progression and treatment response of patients, using the rich new personalized data that are now routinely extracted. The traditional medical statistics and survival analysis methods were created for answering clinical questions of a previous era. They tend to extract only those statistical regularities that are uniform across patient cohorts, predict using only baseline information (disregarding subsequent treatment choices and intermediate responses), disregard interference of further pathologies on the primary cancer, and sidestep causal questions by focusing on associations and correlations, avoiding counterfactual analyses that might have supported and elucidated therapeutic decision making. These limitations are no longer acceptable in post-genome personalized cancer medicine, and require new data analysis tools. The full promise of more powerful methods and richer data sources in personalized cancer outcome and treatment response prediction has not yet been realized, because it requires that we map and tackle one by one the relevant new technical data-related and data-based decision-related challenges to be overcome, and neutralize the danger of overfitting associated with high data dimensionality and model complexity.
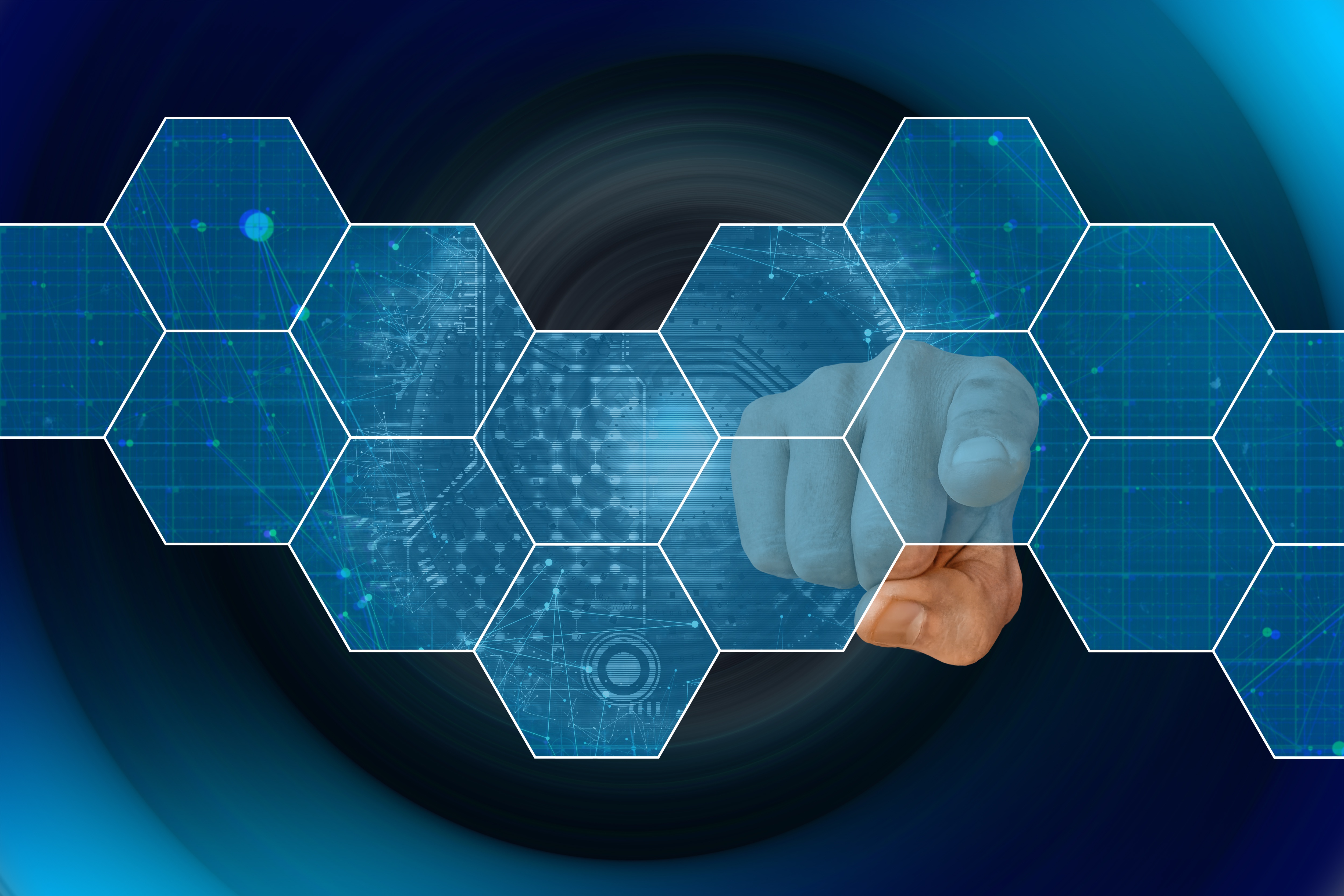
These are the challenges we address in this programme in the following work packages:
2.1 Personalized health care: Radboud University; PI Tom Claassen; and TNO; PI Jildau Bouwman; joint PhD vacancy (industrial doctorate).
2.2 Interacting risks and co-morbidities; Radboud University; PI Ton Coolen; PhD candidate Mauricio Diaz-Ortiz.